AI: Design Ethics vs. End User Ethics — the Difference Is Important
There is currently a flurry of interest in AI ethics. The Pentagon is wrestling with establishing AI ethics for military use. Know-it-all Google is offering its advice on dealing with the tricky ethics of AI.
When examining AI ethics problems, we can distinguish between design ethics and end-user ethics. Design ethics concerns the final performance of an AI product. End-user ethics concerns how the designed AI is used.
Assuring that AI weapons work as desired is the aim of design ethics. Moral concern such as opposition to autonomous AI military weapons that kill is an example of end-user ethics concerns. Design ethics is always the responsibility of the AI programmers and system testers. End-user ethics addresses how the technology is to be used.
Legal language can be applied to the vetting of AI design standards. Some AI makes many mistakes, like Alexa’s response to audio requests to play Spotify tunes. But they are mistakes we can live with. A legal standard here might be that there is a “preponderance of evidence” that Alexa works. Self-driving cars are a more serious matter. Before considering riding in a self-driving car, I would like to know that the vehicle operates as intended “beyond a reasonable doubt.” Total certainty is, unfortunately, never possible.
Measuring the different levels of design assurance is the task of those making regulatory policy and standards. How are “preponderance of evidence” and “beyond a reasonable doubt” quantified? There is much from the field of reliability engineering that can be applied here.
Is biased AI ethical? A headline from Wired reads ”AI Is Biased. Here’s How Scientists Are Trying to Fix It.” In the most basic sense, all software, including AI, is infused with bias. How can a computer add numbers without being biased toward accepting the fundamentals of arithmetic? Without the guiding bias of the programmer, computer programs can do nothing. AI without bias is like ice cubes without cold.
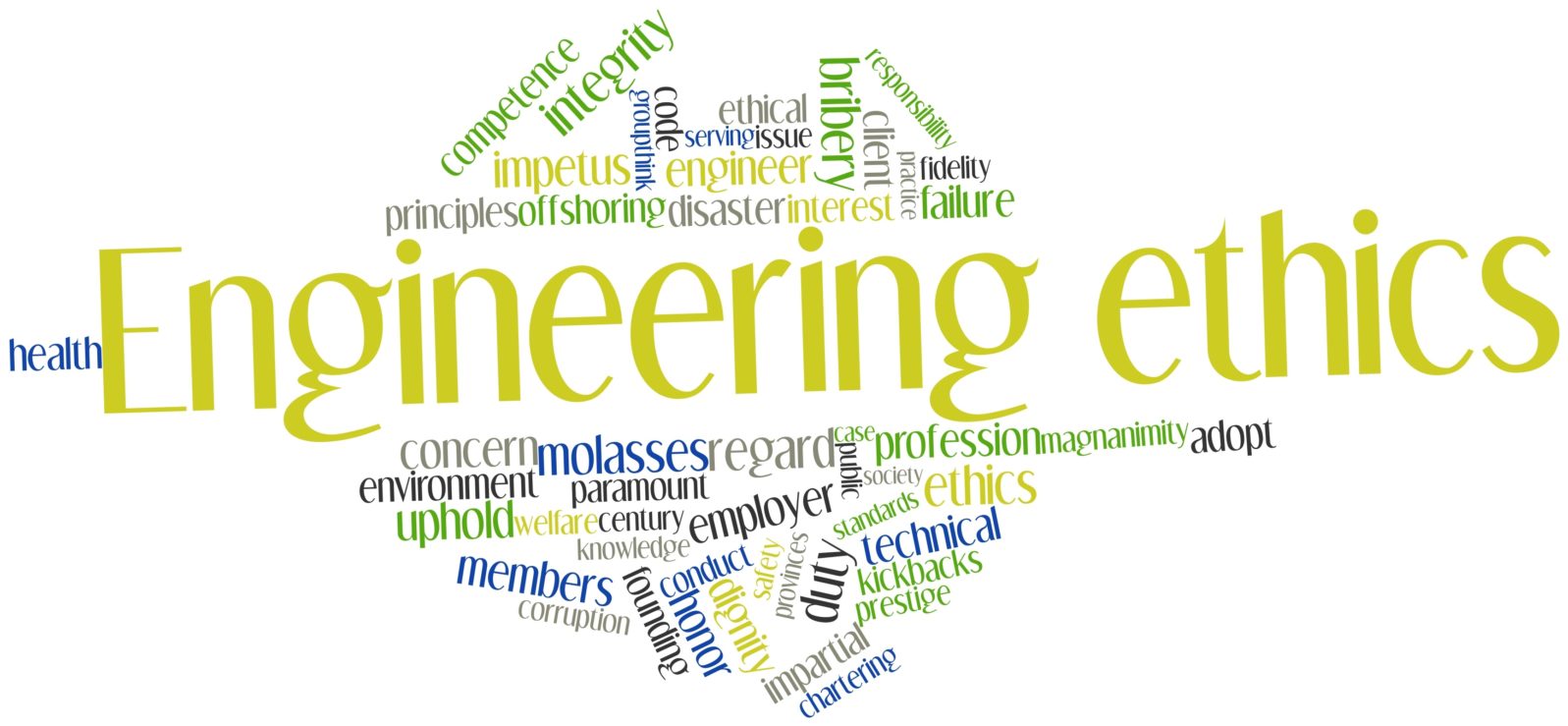
Perceived bias can be resident in the data the AI is analyzing. If data shows that hiring practices were discriminatory in the past, that’s what the AI should announce. Design ethics have been met. If these accurate AI results are found to be unacceptable, the job of the end-user is interpreting the AI results to adjust future hiring practices to conform to current standards.
As I discuss in my book, The Case for Killer Robots, the use of autonomous weapons should not be argued from a design ethics point of view. If an autonomous weapon has passed design scrutiny, it will perform as intended.
Autonomous anti-radiation weapons like Israel’s HARPY missile have been tested to assure that they perform the task they were designed to do. They have been in use for over fifteen years. In such cases, whether or not autonomous weapons should be banned is not a design ethics matter but an end-user debate.
The major ethical challenge in AI design is unintended consequences. An example of a failed design is the Uber self-driving car that killed a pedestrian in 2018. Another is the Google image recognition system that mistakenly labeled pictures of black persons as gorillas. Such unethical AI design acts are not the responsibility of the AI but of the AI programmers and testers.
IEEE, the world’s largest professional society of computer scientists and electrical engineers, has a code of ethics. While the code was being drafted, there had been end-user pressure to include a clause saying that no IEEE member shall contribute to any technology that kills. A gaggle of IEEE engineers (engineers come in “gaggles”) working for defense contractors protested. The mission of the US military necessitates killing, which would mean that this set of IEEE members would be in violation of their professional society’s ethics policy. The proposed addition to the code of ethics was abandoned. Currently, the code asks IEEE members “to hold paramount the safety, health, and welfare of the public.” Participation in conflicts such as just wars complies with this ideological clause.
In establishing AI design ethics policy, end-user ideology and politics should be set aside. All factions of society can participate in the debate but design ethics must focus solely on the quality of the end product. The question as to whether autonomous AI military weapons should be used is largely political and, some would claim, moral. But these end-user concerns have nothing to do with design ethics.
AI with broad goals, like self-driving cars, features an exponential increase in the number of possible design contingencies with respect to a linear increase in complexity. Complying with AI ethical design standards becomes more difficult because vetting becomes more difficult. The problem can be partially mitigated by intense scrutiny and the application of deep domain expertise during the software development and AI system testing. Still, generally, the more complex the AI system, the more difficult the vetting.
The takeaway is this: End-user concerns can drive design specifications and design limitations can impact the end use of AI technology. But design and end-user AI ethics are distinct. One belongs in a research and development lab. The other in the arena of debate and ideas. Both are important considerations but they should not be conflated.
More by Robert J. Marks on AI and ethics:
What’s to be done about cheating with Chegg in the Covid era? College-level solutions to specific problems can be texted, for a fee, to students writing exams.
and
AI ethics and the value of human life Unanticipated consequences will always be a problem for totally autonomous AI.